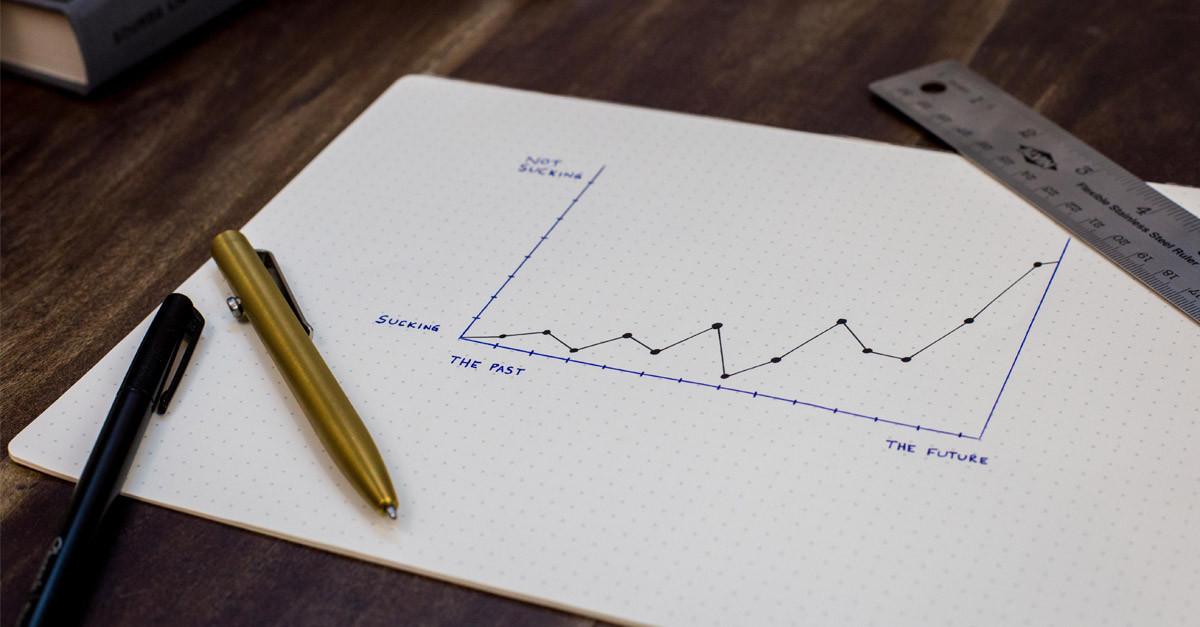
Especially in times of market leaders such as Amazon and Co., companies have to fight over every single customer in order to survive in a highly competitive market. Almost more important than the acquisition of new customers, however, is the retention of existing customers. While approaching new customers causes remarkably high efforts and costs, it is much easier with loyal and satisfied customers, because companies can concentrate on functioning relationships. They are therefore crucial for good company performance at the end of a year. To achieve high customer satisfaction and loyalty, companies have to offer not only the best service but also a unique and personal customer experience across all channels.
But how do retailers know what really makes customers happy?
For retail companies, data analysis should be at the heart of their strategy. Because only those who use and connect data correctly will have the chance to gain a full understanding of their customers. The prerequisite for sustainable business success is, therefore, in addition to a complete and high-quality database, a solution for structured data analysis.
In order to understand which levers can be used to control customer satisfaction and what this means for a company’s success, you have to analyze company data systematically on a regular basis. One challenge is the multitude of isolated data silos that can be found in every organization. The data is either not evaluated at all or only “one-dimensionally”. This leads to incomplete or even wrong interpretation of the key performance drivers. The ultimate goal has to be to break down these data silos in order to provide a central data basis for a company-wide analysis.
The data can be transactional, product data, customer data, and campaign data. The NPS, for example, is an important key figure that provides information on customer satisfaction, rounds off the holistic customer picture, and should therefore not be missing in the analysis. However, it is not always sufficient to know which customer has a high NPS, rather it is essential to find out what a high NPS means in monetary terms for customer value. In order to achieve this, all data sources must be compiled in one system and connected in order to form a consistent picture.
At first, the data has to be standardized so that it is accessible to every business user for the most intuitive actions. This could be a comprehensive project for your own IT or you can take the faster route via a standard solution that is established on the market and that provides the necessary know-how and technical setup.
The complete understanding of the customer
By integrating various data sources (online and offline), retailers get full Omni-Channel transparency and can not only track the customer’s journey until the purchase but also understand what happens after the transaction. Which product category leads to the highest number of returns and why do customers return these products? How does customer lifetime value behave and therefore affect profitability for loyal customers? How do new and existing customers develop through different channels? What cross- and up-selling potential do satisfied customers have? And how much money is it worth investing in less satisfied customers, to improve example given their NPS?
Retailers can therefore understand precisely which factors (products, fulfillment, time-on-site, website performance, etc.) influence customer satisfaction. They can recognize for example, what impact these parameters have on the value of a customer (CLV) and which (marketing) measures can be used to increase this value and ultimately boost sales.
With an appropriate solution for data analysis, companies can also build flexible customer segments directly and address customers individually with relevant offers. For example, they can offer well-converting cross-selling products in a campaign. Even customers who are at risk of churn can be addressed with automated next best offer campaigns when they deviate from their natural behavior (e.g. long periods of inactivity). If automation is used strategically, not only can the CLV be raised significantly, but in addition to customer loyalty and process efficiency, customer satisfaction and revenue can also be increased.
Conclusion: The relevant data is available in most cases, but few people can use it. Therefore, a suitable solution for company-wide, intelligent data analysis is needed. This should enable every employee to access relevant data autonomously without the need for technical knowledge. Retailers with a data-driven work culture gain a complete understanding of their customers and are able to make both strategic and operational decisions more efficient, thus improving the buying experience, service, and ultimately the company’s performance.
Tags: Bylines & Interviews